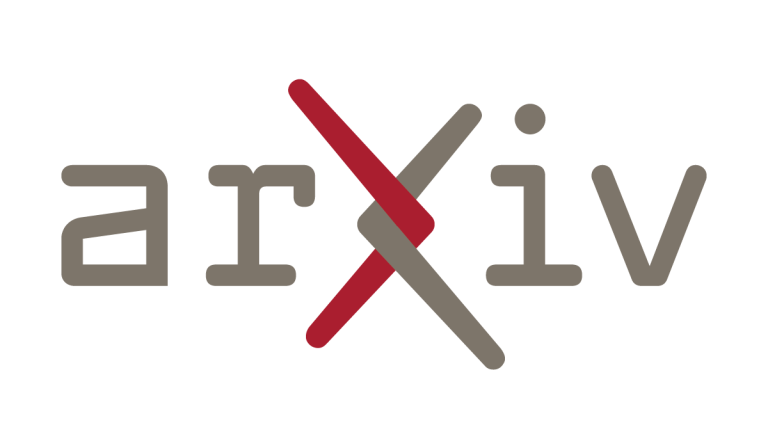
[ad_1]
Obtain a PDF of the paper titled The committee machine: Computational to statistical gaps in studying a two-layers neural community, by Benjamin Aubin and 4 different authors
Summary:Heuristic instruments from statistical physics have been used previously to find the section transitions and compute the optimum studying and generalization errors within the teacher-student state of affairs in multi-layer neural networks. On this contribution, we offer a rigorous justification of those approaches for a two-layers neural community mannequin referred to as the committee machine. We additionally introduce a model of the approximate message passing (AMP) algorithm for the committee machine that permits to carry out optimum studying in polynomial time for a big set of parameters. We discover that there are regimes by which a low generalization error is information-theoretically achievable whereas the AMP algorithm fails to ship it, strongly suggesting that no environment friendly algorithm exists for these circumstances, and unveiling a big computational hole.
Submission historical past
From: Antoine Maillard [view email]
[v1]
Thu, 14 Jun 2018 10:22:04 UTC (127 KB)
[v2]
Fri, 14 Jun 2019 15:34:07 UTC (485 KB)
[v3]
Thu, 29 Feb 2024 11:10:45 UTC (142 KB)
[ad_2]
Supply hyperlink