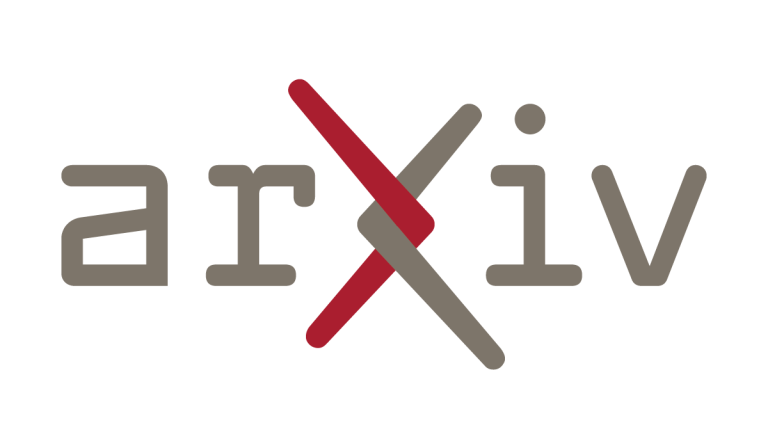
[ad_1]
Obtain a PDF of the paper titled A Basic Principle for Kernel Packets: from state house mannequin to compactly supported foundation, by Liang Ding and Rui Tuo
Summary:It’s well-known that the state house (SS) mannequin formulation of a Gaussian course of (GP) can decrease its coaching and prediction time each to O(n) for n information factors. We show that an $m$-dimensional SS mannequin formulation of GP is equal to an idea we introduce as the final proper Kernel Packet (KP): a metamorphosis for the GP covariance operate $Ok$ such that $sum_{i=0}^{m}a_iD_t^{(j)}Ok(t,t_i)=0$ holds for any $t leq t_1$, 0 $leq j leq m-1$, and $m+1$ consecutive factors $t_i$, the place ${D}_t^{(j)}f(t) $ denotes $j$-th order spinoff appearing on $t$. We lengthen this concept to the backward SS mannequin formulation of the GP, resulting in the idea of the left KP for subsequent $m$ consecutive factors: $sum_{i=0}^{m}b_i{D}_t^{(j)}Ok(t,t_{m+i})=0$ for any $tgeq t_{2m}$. By combining each left and proper KPs, we will show {that a} appropriate linear mixture of those covariance features yields $m$ compactly supported KP features: $phi^{(j)}(t)=0$ for any $tnotin(t_0,t_{2m})$ and $j=0,cdots,m-1$. KPs additional cut back the prediction time of GP to O(log n) and even O(1), may be utilized to extra basic issues involving the spinoff of GPs, and have multi-dimensional generalization for scattered information.
Submission historical past
From: Liang Ding [view email]
[v1]
Tue, 6 Feb 2024 14:12:46 UTC (702 KB)
[v2]
Wed, 7 Feb 2024 18:36:18 UTC (2,249 KB)
[v3]
Thu, 8 Feb 2024 07:56:25 UTC (2,249 KB)
[ad_2]
Supply hyperlink