As synthetic intelligence (AI) continues to evolve, its deployment has expanded past cloud computing into edge gadgets, bringing transformative benefits to numerous industries.
AI inference on the edge computing refers back to the technique of operating educated AI fashions instantly on native {hardware}, reminiscent of smartphones, sensors, and IoT gadgets, moderately than counting on distant cloud servers for knowledge processing.
This fast evolution of the know-how panorama with the convergence of synthetic intelligence (AI) and edge computing represents a transformative shift in how knowledge is processed and utilized.
This shift is revolutionizing how real-time knowledge is analyzed, providing unprecedented advantages when it comes to velocity, privateness, and effectivity. This synergy brings AI capabilities nearer to the supply of information technology, unlocking new potential for real-time decision-making, enhanced safety, and effectivity.
This text delves into the advantages of AI inference in edge computing and explores numerous use instances throughout completely different industries.
Fig 1. Advantages of AI Inference in edge computing
Actual-time processing
One of the crucial important benefits of AI inference on the edge is the power to course of knowledge in real-time. Conventional cloud computing typically entails sending knowledge to centralized servers for evaluation, which may introduce latency as a result of distance and community congestion.
Edge computing mitigates this by processing knowledge domestically on edge gadgets or close to the information supply. This low-latency processing is essential for purposes requiring fast responses, reminiscent of autonomous autos, industrial automation, and healthcare monitoring.
Privateness and safety
Transmitting delicate knowledge to cloud servers for processing poses potential safety dangers. Edge computing addresses this concern by holding knowledge near its supply, lowering the necessity for in depth knowledge transmission over probably susceptible networks.
This localized processing enhances knowledge privateness and safety, making edge AI significantly precious in sectors dealing with delicate data, reminiscent of finance, healthcare, and protection.
Bandwidth effectivity
By processing knowledge domestically, edge computing considerably reduces the quantity of information that must be transmitted to distant cloud servers. This discount in knowledge transmission necessities has a number of necessary implications; it ends in diminished community congestion, because the native processing on the edge minimizes the burden on community infrastructure.
Secondly, the diminished want for in depth knowledge transmission results in decrease bandwidth prices for organizations and end-users, as transmitting much less knowledge over the Web or mobile networks can translate into substantial financial savings.
This profit is especially related in environments with restricted or costly connectivity, reminiscent of distant places. In essence, edge computing optimizes the utilization of obtainable bandwidth, enhancing the general effectivity and efficiency of the system.
Scalability
AI methods at edge will be scaled effectively by deploying extra edge gadgets as wanted, with out overburdening central infrastructure. This decentralized strategy additionally enhances system resilience. Within the occasion of community disruptions or server outages, edge gadgets can proceed to function and make selections independently, making certain uninterrupted service.
Power effectivity
Edge gadgets are sometimes designed to be energy-efficient, making them appropriate for environments the place energy consumption is a essential concern. By performing AI inference domestically, these gadgets reduce the necessity for energy-intensive knowledge transmission to distant servers, contributing to total power financial savings.
{Hardware} accelerator
AI accelerators, reminiscent of NPUs, GPUs, TPUs, and customized ASICs, play a essential function in enabling environment friendly AI inference on the edge. These specialised processors are designed to deal with the intensive computational duties required by AI fashions, delivering excessive efficiency whereas optimizing energy consumption.
By integrating accelerators into edge gadgets, it turns into attainable to run complicated deep studying fashions in actual time with minimal latency, even on resource-constrained {hardware}. This is among the greatest enablers of AI, permitting bigger and extra highly effective fashions to be deployed on the edge.
Offline operation
Offline operation via Edge AI in IoT is a essential asset, significantly in eventualities the place fixed web connectivity is unsure. In distant or inaccessible environments the place community entry is unreliable, Edge AI methods guarantee uninterrupted performance.
This resilience extends to mission-critical purposes, enhancing response occasions and lowering latency, reminiscent of in autonomous autos or safety methods. Edge AI gadgets can domestically retailer and log knowledge when connectivity is misplaced, safeguarding knowledge integrity.
Moreover, they function an integral a part of redundancy and fail-safe methods, offering continuity and decision-making capabilities, even when major methods are compromised. This functionality augments the adaptability and dependability of IoT purposes throughout a large spectrum of operational settings.
Customization and personalization
AI inference on the edge allows a excessive diploma of customization and personalization by processing knowledge domestically, permitting methods to deploy personalized fashions for particular person person wants and particular environmental contexts in real-time.
AI methods can rapidly reply to modifications in person habits, preferences, or environment, providing extremely tailor-made providers. The power to customise AI inference providers on the edge with out counting on steady cloud communication ensures sooner, extra related responses, enhancing person satisfaction and total system effectivity.
The normal paradigm of centralized computation, whereby these fashions reside and function solely inside knowledge facilities, has its limitations, significantly in eventualities the place real-time processing, low latency, privateness preservation, and community bandwidth conservation are essential.
This demand for AI fashions to course of knowledge in actual time whereas making certain privateness and effectivity has given rise to a paradigm shift for AI inference on the edge. AI researchers have developed numerous optimization strategies to enhance the effectivity of AI fashions, enabling AI mannequin deployment and environment friendly inference on the edge.
Within the subsequent part we’ll discover a few of the use instances of AI inference utilizing edge computing throughout numerous industries.
The fast developments in synthetic intelligence (AI) have reworked quite a few sectors, together with healthcare, finance, and manufacturing. AI fashions, particularly deep studying fashions, have confirmed extremely efficient in duties reminiscent of picture classification, pure language understanding, and reinforcement studying.
Performing knowledge evaluation instantly on edge gadgets is changing into more and more essential in eventualities like augmented actuality, video conferencing, streaming, gaming, Content material Supply Networks (CDNs), autonomous driving, the Industrial Web of Issues (IoT), clever energy grids, distant surgical procedure, and security-focused purposes, the place localized processing is important.
On this part, we’ll talk about use instances throughout completely different fields for AI inference on the edge, as proven in Fig 2.
Fig 1. Purposes of AI Inference on the Edge throughout completely different fields
Web of Issues (IoT)
The growth of the Web of Issues (IoT) is considerably pushed by the capabilities of sensible sensors. These sensors act as the first knowledge collectors for IoT, producing giant volumes of data.
Nonetheless, centralizing this knowledge for processing may end up in delays and privateness points. That is the place edge AI inference turns into essential. By integrating intelligence instantly into the sensible sensors, AI fashions facilitate fast evaluation and decision-making proper on the supply.
This localized processing reduces latency and the need to ship giant knowledge portions to central servers. In consequence, sensible sensors evolve from mere knowledge collectors to real-time analysts, changing into important within the progress of IoT.
Industrial purposes
In industrial sectors, particularly manufacturing, predictive upkeep performs a vital function in figuring out potential faults and anomalies in processes earlier than they happen. Historically, heartbeat indicators, which replicate the well being of sensors and equipment, are collected and despatched to centralized cloud methods for AI evaluation to foretell faults.
Nonetheless, the present development is shifting. By leveraging AI fashions for knowledge processing on the edge, we are able to improve the system’s efficiency and effectivity, delivering well timed insights at a considerably diminished price.
Cell / Augmented actuality (AR)
Within the discipline of cell and augmented actuality, the processing necessities are important as a result of must deal with giant volumes of information from numerous sources reminiscent of cameras, Lidar, and a number of video and audio inputs.
To ship a seamless augmented actuality expertise, this knowledge have to be processed inside a stringent latency vary of about 15 to twenty milliseconds. AI fashions are successfully utilized via specialised processors and cutting-edge communication applied sciences.
The mixing of edge AI with cell and augmented actuality ends in a sensible mixture that enhances real-time evaluation and operational autonomy on the edge. This integration not solely reduces latency but additionally aids in power effectivity, which is essential for these quickly evolving applied sciences.
Safety methods
In safety methods, the mixture of video cameras with edge AI-powered video analytics is remodeling risk detection. Historically, video knowledge from a number of cameras is transmitted to cloud servers for AI evaluation, which may introduce delays.
With AI processing on the edge, video analytics will be carried out instantly inside the cameras. This permits for fast risk detection, and relying on the evaluation’s urgency, the digicam can rapidly notify authorities, lowering the possibility of threats going unnoticed. This transfer to AI-integrated safety cameras improves response effectivity and strengthens safety at essential places reminiscent of airports.
Robotic surgical procedure
In essential medical conditions, distant robotic surgical procedure entails conducting surgical procedures with the steering of a surgeon from a distant location. AI-driven fashions improve these robotic methods, permitting them to carry out exact surgical duties whereas sustaining steady communication and path from a distant medical skilled.
This functionality is essential within the healthcare sector, the place real-time processing and responsiveness are important for easy operations below high-stress situations. For such purposes, it is important to deploy AI inference on the edge to make sure security, reliability, and fail-safe operation in essential eventualities.
Pc imaginative and prescient meets robotics: the way forward for surgical procedure
Max Allan, Senior Pc Imaginative and prescient Engineer at Intuitive, describes groundbreaking robotics improvements in surgical procedure and the healthcare business.
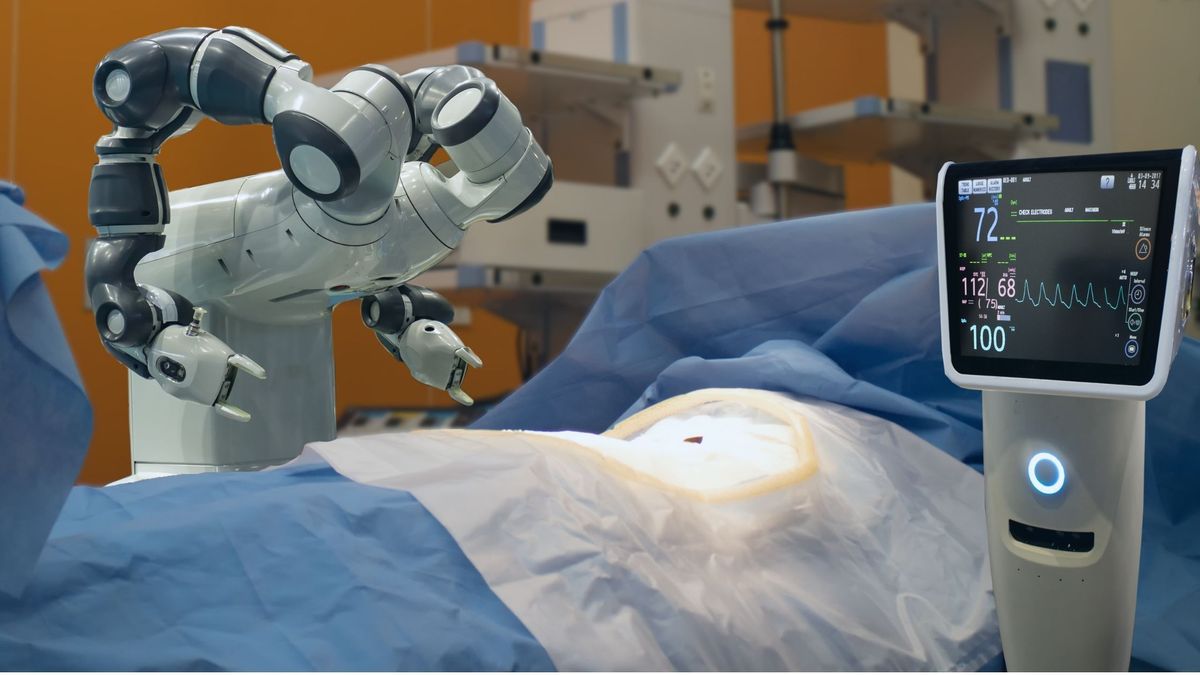
Autonomous driving
Autonomous driving is a pinnacle of technological progress, with AI inference at edge taking a central function. AI accelerators within the automobile empower autos with onboard fashions for fast real-time decision-making.
This fast evaluation allows autonomous autos to navigate complicated eventualities with minimal latency, bolstering security and operational effectivity. By integrating AI on the edge, self-driving automobiles adapt to dynamic environments, making certain safer roads and diminished reliance on exterior networks.
This fusion represents a transformative shift, the place autos grow to be clever entities able to swift, localized decision-making, ushering in a brand new period of transportation innovation.
The mixing of AI inference in edge computing is revolutionizing numerous industries by facilitating real-time decision-making, enhancing safety, and optimizing bandwidth utilization, scalability, and power effectivity.
As AI know-how progresses, its purposes will broaden, fostering innovation and rising effectivity throughout numerous sectors. The benefits of edge AI are evident in fields such because the Web of Issues (IoT), healthcare, autonomous autos, and cell/augmented actuality gadgets.
These applied sciences profit from the localized processing that edge AI allows, promising a future the place clever, on-the-spot analytics grow to be the usual. Regardless of the promising developments, there are ongoing challenges associated to the accuracy and efficiency of AI fashions deployed on the edge.
Guaranteeing that these methods function reliably and successfully stays a essential space of analysis and improvement. The widespread adoption of edge AI throughout completely different fields highlights the pressing want to deal with these challenges, making strong and environment friendly edge AI deployment a brand new norm.
As analysis continues and know-how evolves, the potential for edge AI to drive important enhancements in numerous domains will solely develop, shaping the way forward for clever, decentralized computing.
Wish to know extra about how generative firms are utilizing AI?
Get your copy of our Gen AI report beneath!
Generative AI 2024 report
Unlock the secrets and techniques to sooner workflows with the Generative AI 2024 Report. Find out how 56.4% of firms leverage AI to spice up effectivity and keep aggressive.
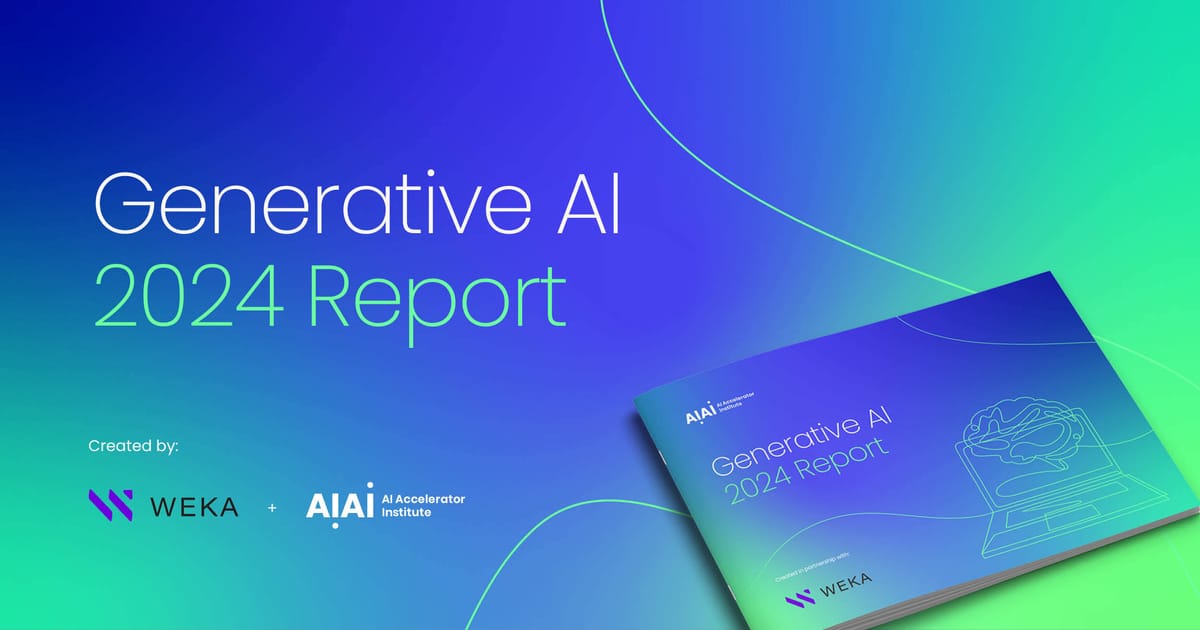