Organizations of all sizes are racing to deploy generative AI to assist drive total effectivity and take away prices from their companies. As with every new expertise, deployment usually comes with boundaries that may stall progress and implementation timelines.
LXT’s newest AI maturity report displays the views of 315 executives working in AI and divulges the highest bottlenecks firms face when deploying generative AI.
These embody:
- Safety and privateness considerations
- Accuracy of the output of the answer
- Availability of high quality coaching knowledge
- Tremendous-tuning the foundational mannequin
- Immediate tuning
- Accuracy of foundational fashions
Only one% of respondents said they weren’t experiencing any bottlenecks with their generative AI deployments.
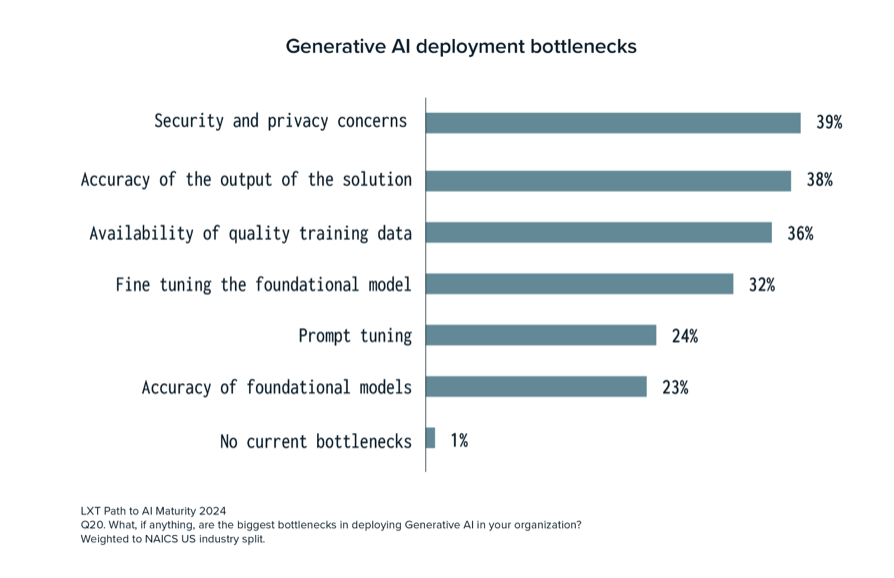
1. Safety and privateness considerations
39% of respondents highlighted safety and privateness considerations as their prime bottleneck in deploying generative AI. This isn’t shocking as generative AI fashions require a considerable amount of coaching knowledge. Firms should guarantee correct knowledge governance to keep away from exposing private knowledge and delicate info, reminiscent of names, addresses, contact particulars, and even medical information.
Further safety considerations for generative AI fashions embody adversarial assaults the place unhealthy actors trigger the fashions to generate inaccurate and even dangerous outputs. Lastly, care should be taken to make sure that generative AI fashions don’t generate content material that mimics mental property which might result in authorized scorching water.
Firms can mitigate these dangers by acquiring consent from anybody whose knowledge is getting used to coach its generative AI fashions, just like how consent is obtained when utilizing people’ pictures on web sites. Further knowledge governance procedures must be applied as properly, together with knowledge retention insurance policies and redacting personally identifiable info (PII) to keep up particular person confidentiality.
2. Accuracy of the output of the answer
Neck and neck with safety and privateness considerations, 38% of respondents said that the accuracy of generative AI’s output is a prime problem. We’ve all seen the information articles about chatbots spewing out misinformation and even going rogue.
Whereas generative AI has immense potential to streamline enterprise processes, it wants guardrails to remove hallucinations, guarantee accuracy, and preserve buyer belief. For instance, firms ought to have 100% readability on the supply and accuracy of the information getting used to coach their fashions and will preserve documentation on these sources.
Additional, human-in-the-loop processes for evaluating the accuracy of coaching knowledge, in addition to the output of generative AI methods, are essential. This could additionally assist scale back knowledge bias in order that generative AI fashions function as supposed.
3. Availability of high quality coaching knowledge
Excessive-quality coaching knowledge is important, because it immediately impacts AI fashions’ reliability, efficiency, and accuracy. It permits fashions to make higher selections and create extra reliable outcomes.
36% of respondents in LXT’s survey said that the provision of high-quality coaching knowledge is a problem with generative AI deployments. Latest press even has highlighted that human-written textual content could possibly be used up for chatbot coaching by 2023. This bottleneck prevents firms from having the ability to scale their fashions effectively, which then impacts the standard of their output.
In relation to deploying any AI answer, the information wanted to coach the fashions must be handled as a person product with its personal lifecycle. Firms must be deliberate about planning for the quantity and kind of information they should assist the lifecycle of their AI answer. A knowledge providers associate can present steerage on knowledge options that can create optimum outcomes.
4. Tremendous-tuning the foundational mannequin
Tremendous-tuning contains bettering open-source, pre-trained basis fashions, which frequently incorporate educational fine-tuning, reinforcement studying with human or AI suggestions (RLHR/RLAIF), or domain-specific pre-training.
32% of respondents said that fine-tuning the foundational mannequin could be a problem when deploying generative AI, because it requires a deep understanding of enormous basis mannequin (LFM) coaching enhancements and transformer fashions. To fine-tune fashions, firms should even have staff who can velocity up coaching processes utilizing instruments for multi-machine coaching and multi-GPU setups, for instance.
Nonetheless, regardless of its potential good points, fine-tuning has a number of points. Submit-training inaccuracies can improve, the mannequin may be overloaded with giant domain-specific corpora and have minimized generalization capacity, and extra.
To fight potential points in fine-tuning fashions, reinforcement studying and supervised fine-tuning are helpful strategies, as they assist to take away dangerous info and bias from responses that LLMs generate.
5. Immediate tuning
Immediate tuning is a way that adjusts the prompts that inform a pre-trained language mannequin’s response with out a full overhaul of its weights. These prompts are built-in right into a mannequin’s enter processing. 24% of respondents said that immediate tuning presents challenges when deploying generative AI.
Immediate tuning is only one methodology that can be utilized to enhance an LLM’s efficiency on a job. Tremendous-tuning and immediate engineering are different strategies that can be utilized to enhance mannequin efficiency, and every methodology varies by way of assets wanted and coaching required.
Within the case of immediate tuning, this methodology is finest suited to sustaining a mannequin’s integrity throughout duties. It doesn’t require as many computational assets as fine-tuning and doesn’t require as a lot human involvement in comparison with immediate engineering.
Firms deploying generative AI ought to consider their use case to find out one of the best ways to enhance their language fashions.
6. Accuracy of foundational fashions
23% of respondents in LXT’s survey stated that the accuracy of foundational fashions is a problem of their generative AI deployments. Foundational fashions are pre-trained to carry out a spread of duties and are used for pure language processing, pc imaginative and prescient, speech processing, and extra.
Foundational fashions present firms with instant entry to high quality knowledge with out having to spend time coaching their mannequin and with out having to take a position as closely in knowledge science assets.
There are some challenges with these fashions, nevertheless, together with lack of accuracy and bias. If the mannequin is just not skilled on a various dataset, it received’t be inclusive of the inhabitants at giant and will lead to AI options that alienate sure demographic teams. It’s crucial for organizations utilizing these fashions to grasp how they had been skilled and tune them for higher accuracy and inclusiveness.
Get extra insights within the full report
With the quickly evolving area of AI, preserving up-to-date with the developments and developments is important for achievement in AI initiatives. LXT’s Path to AI Maturity report offers you a present image of the state of AI maturity within the enterprise, the quantity of funding made, the highest use circumstances for generative AI, and rather more.
Obtain the report at this time to entry the total analysis findings.